How Predictive Analytics Gets Nurse Staff Forecasting Wrong
In the complex world of healthcare staffing, the balance between supply and demand is delicate. Predictive analytics has emerged as a powerful tool to forecast staffing needs, but it often falls short in one critical area: distinguishing between the different types of nurse labor. Traditional forecasting models tend to overlook the unique characteristics of contingent labor, such as per diem and traveler nurses, leading to inefficiencies and increased costs.
Demand vs. Supply: Understanding the Nuances
To grasp why predictive analytics often fails in nurse staff forecasting, it’s essential to differentiate between demand and supply in healthcare settings. Demand is driven by patient volume and acuity mix—the severity and types of conditions with which patients present. This creates a dynamic and often unpredictable workload that hospitals must manage. On the other hand, supply is about having the right number of nurses with the appropriate skills and specialties available to meet that demand.
Contingent labor, plays a crucial role in this equation. These nurses provide flexibility and can fill gaps quickly, but they also come with higher costs compared to regular staff. Despite their importance, most forecasting models treat all nurse labor as homogeneous, failing to account for the differences between regular and contingent staff.
The Shortcomings of Traditional Predictive Models
A common flaw in many predictive analytics models is the assumption that all nurses are interchangeable. These models typically analyze historical data on scheduled and filled shifts to predict future needs. However, they often neglect the context of these shifts—specifically, the ratio of regular staff to contingent labor.
Consider a hospital that has historically filled its shifts with a 10:1 ratio of regular to contingent nurses. If the forecasting model only looks at the number of shifts filled and not the type of labor used, it might predict future needs without accounting for the cost implications. Now, imagine another scenario where the ratio was 5:1. The labor costs in this scenario would be significantly higher due to the increased reliance on more expensive contingent nurses. Without incorporating these nuances, predictions can lead to over-reliance on contingent labor, driving up costs unnecessarily.
A Holistic Approach: Integrating Demand and Supply Optimization
To address these shortcomings, healthcare systems need a more sophisticated approach that integrates both demand forecasting and supply optimization. This means not only predicting the number of shifts needed but also strategically planning the mix of staffing types to fulfill those shifts.
An advanced model should:
- Incorporate Historical Data on Labor Types: By analyzing past data, including the ratios of regular to contingent staff, models can better predict future needs and costs.
- Consider Patient Acuity and Volume Trends: Understanding the specific demands based on patient conditions can help tailor staffing needs more precisely.
- Optimize Staffing Mix: Use predictive insights to plan the most cost-effective mix of regular and contingent labor, ensuring that the right type of nurse is available at the right time.
The Benefits of a Refined Approach
Implementing a more integrated forecasting and optimization model can yield several benefits:
- Cost Reduction: By optimizing the use of regular staff and minimizing reliance on expensive contingent labor, hospitals can significantly reduce staffing costs.
- Improved Quality of Care: Ensuring the right mix of staff can improve patient outcomes, as regular staff typically have more familiarity with hospital protocols and patient needs.
- Nurse Satisfaction: A well-planned staffing strategy can lead to better work environments, reducing burnout and turnover among nurses. Contingent nurses, when used appropriately, can also enjoy more predictable and satisfying work schedules.
Conclusion
Predictive analytics holds great promise for nurse staff forecasting, but it must evolve to address the unique characteristics of contingent labor. By incorporating a more nuanced understanding of supply dynamics and optimizing the mix of staffing types, healthcare systems can achieve better cost efficiency, higher quality care, and improved nurse satisfaction. It’s time to move beyond one-size-fits-all models and embrace a more comprehensive approach to workforce planning.
Share this
You May Also Like
These Related Stories
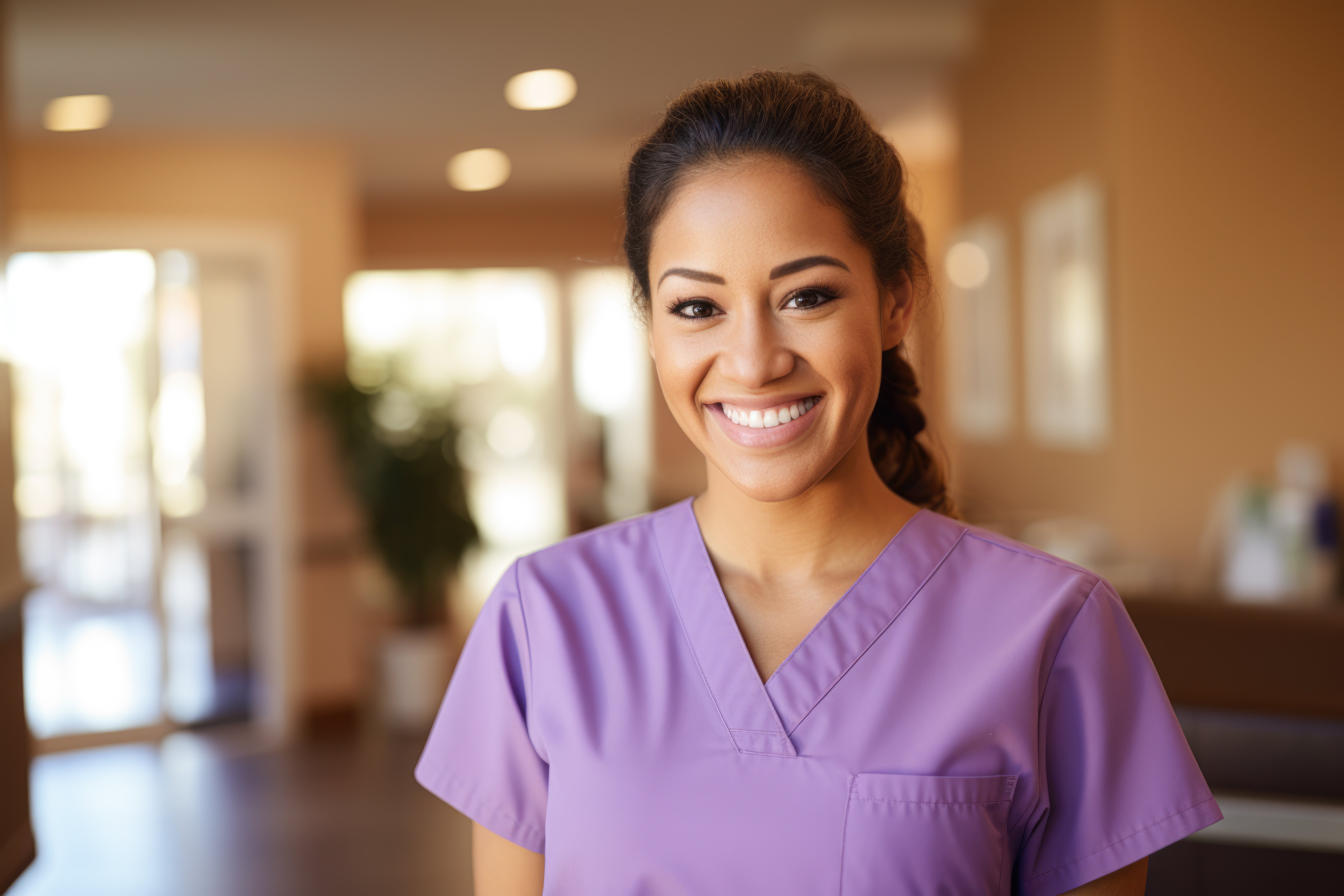
Creating a Supportive Work Environment: A Key Solution for Nurse Retention
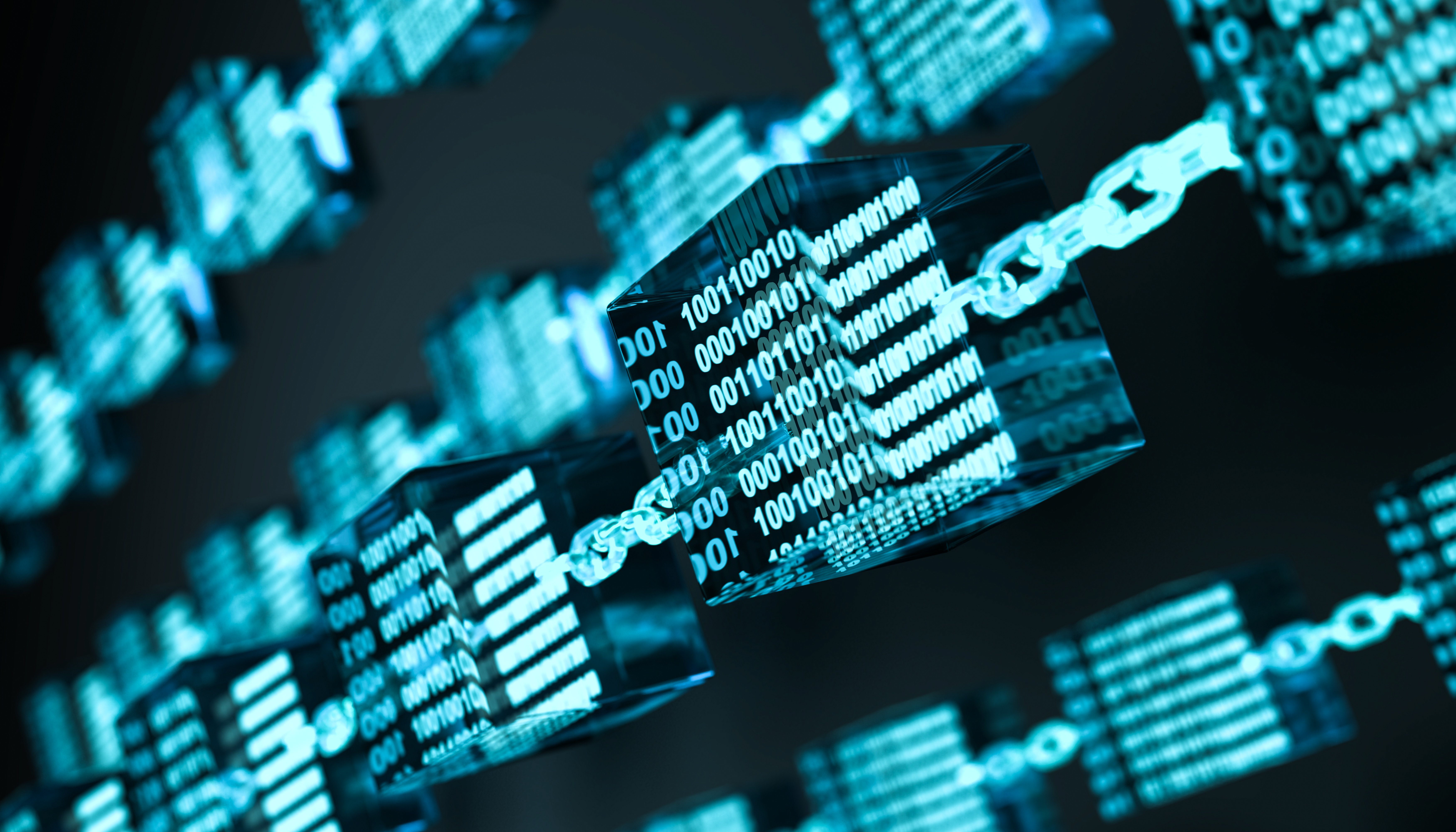
Revolutionizing Credentialing: Blockchain's Role in Nurse Credentialing
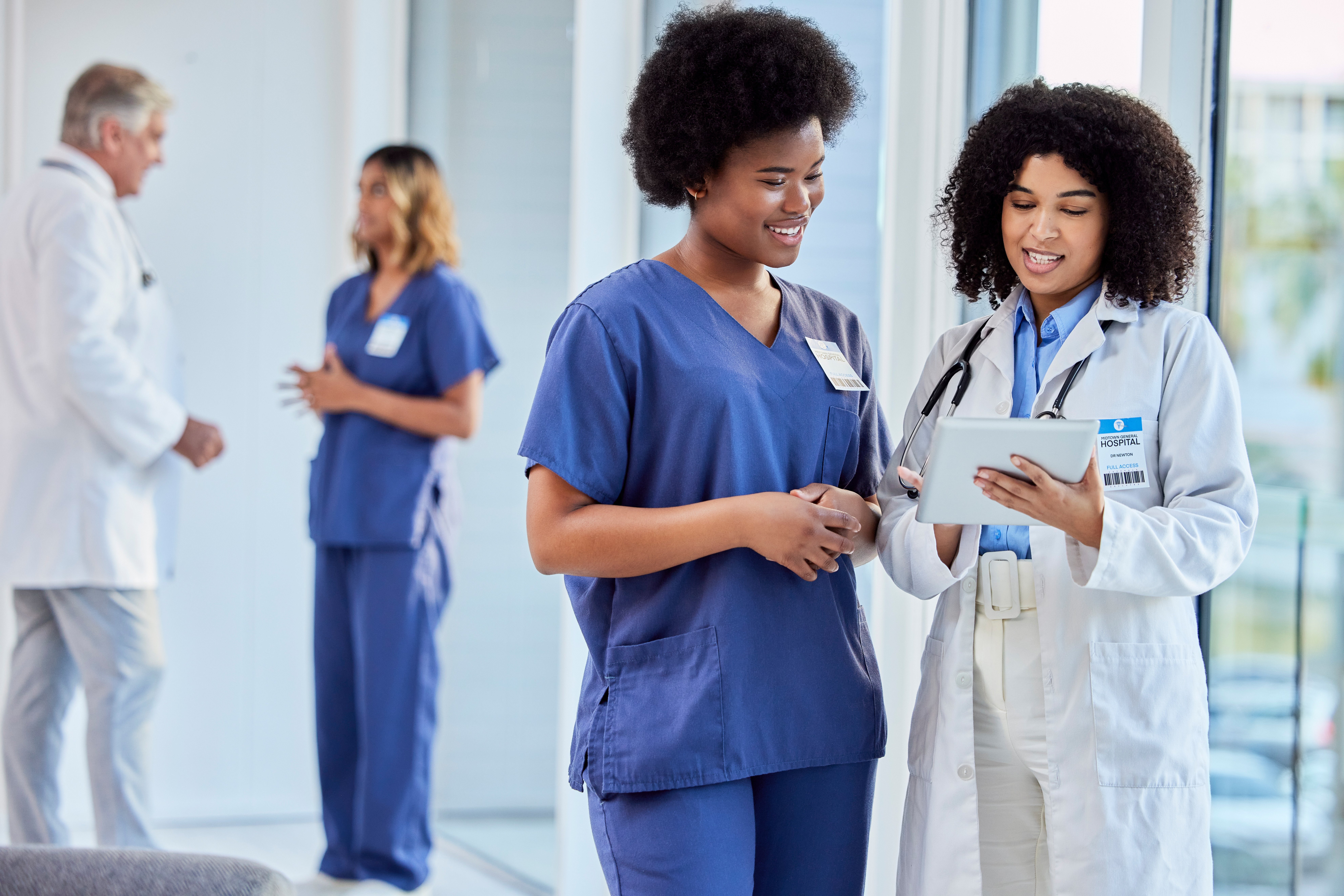